Archive: First steps with Convolutional Networks
Note: Originally posted April 29th, 2021, this is post in the archived Deep Learning for Computer Vision series (cs231n).
- Browse the full cs231n series.
- See the source code in the ConvolutionalNetworks notebook.
New comments are found exclusively in info boxes like this one.
I’ve finished the batchnorm code, and have moved on to the ConvolutionalNetworks.ipynb, which is where the student implements CPU-based convolutional layers and constructs a (very simple) convolutional neural net using some Cython code the author’s prepared for demo purposes (to speed up training epochs).
The convolutional network notes are super helpful here. Additionally, I found this talk (sort of a greatest hits of cs231n) to be extremely useful in grasping the more general idea of ConvNets and the affect they’ve had on the computer vision research space.
Convolutional Neural Networks
Fortunately, I was given a very brief introduction to convolutional nets in some coursework this semester (amusingly, through slides which appear to be largely adapted from the cs231n course material), but it took me a while to understand one of the bigger ‘wins’ of convolution - specifically, that while particular image filters in the past were the result of careful study and domain expertise, that now, you can have a model learn useful filters (i.e. convolutional layer weights) through an automated process. This is an oversimplification, but I feel a useful heuristic/frame-of-reference when starting out.
With at least something resembling an idea of my goal, it was relatively straightforward to implement the naive forward/backward passes the assignment has you start off with (after getting accustomed to working with non-flattened input data). I ran the unit tests and noted the accuracies were all within listed tolerances.
Implementing the 3-layer ConvNet the assignment has you create requires you compile the Cython code shipped with the starter code. After messing about for a bit getting the those extensions to compile properly in my local environment, I was able to compose that in much the same way as the affine layers before. I was able to verify these are working as anticipated by overfitting on a toy dataset of a few samples.
TODO: Implement Spatial/Group batch normalization per the instructions.
Update (2021): Have been reading through both the PyTorch and TensorFlow notebooks to get a general sense of how they differ, as well as looking through instructions to get PyTorch installed on my system (in a sane way). But I’ll finish the ‘from-scratch’-CNN before moving on.
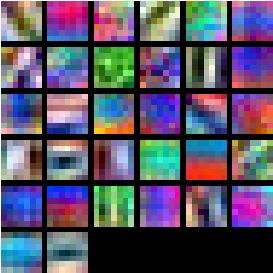
This shows each of our 32 convolutional filters ( ) from the first layer ( ), where each filter has 3 channels, each of size (i.e. ). Since each filter has an immediate visual interpretation, grid visualization an easy way to inspect what’s happening.
We can see some edge detectors are learned for vertical & horizontal edges, among other things. There’s definitely a more recent source, but you can see more about this technique of interpreting CNN weights in this video.